Why LLMs May (Yet) Fall Short of Saving the World
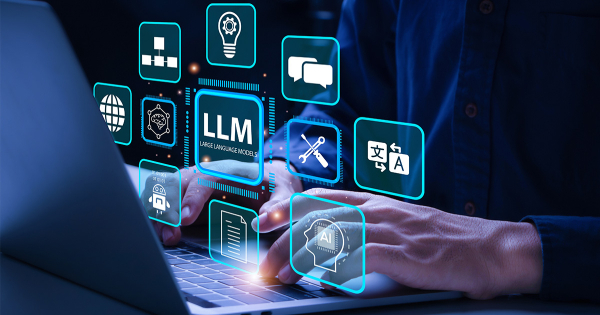
Large Language Models (LLMs) took the world by storm after OpenAI launched its generative pretraining transformer (GPT) engine and debuted ChatGPT in November 2022. In just two months, ChatGPT achieved a significant milestone of 100 million weekly active users, garnering the attention of business and technology leaders across industries.
While a growing body of users today are eager to integrate LLMs into their operations, the technology, in its current avatar, still requires considerable research and development for optimal performance. A recent survey of 150 senior executives from 29 countries revealed that 58% of companies are experimenting with LLMs, and the number only looks set to grow even further – underscoring the need for an accelerated development paradigm.
In a short period, LLMs today have seen broad applications across segments – from customer service automation to test automation and validation. However, the underlying systems, including Natural Language Processing (NLP), continue to face a range of limitations. We explore the boundaries here, and try to identify what the future holds for us.
Beyond the Hype: Exploring the Limitations of LLMs
While LLMs have undeniably captured the imagination of businesses and users worldwide, they are not without some critical limitations. These include:
Data-embedded Biases and Prejudices
LLMs are designed to create a language that feels natural to humans but not necessarily to provide accurate information. This can result in biases and incorrect results if the model is trained on skewed data, resulting in a tendency to “hallucinate” – generate convincing yet factually incorrect output.
Organizations, therefore, need to ensure their models are trained on unbiased data and verify LLM predictions against actual enterprise data.
An example of this was observed in Google’s AI chatbot, Bard, which mistakenly included non-existent discoveries by the James Webb Space Telescope in its responses. This error significantly impacted Google’s stock value, causing a $100 billion loss after being highlighted in a live demonstration. In another instance, ChatGPT was used in a legal case to cite legal precedents that did not exist, highlighting the risks of relying on LLM-generated information without proper verification.
Data Security and Privacy
LLMs learn from vast amounts of data, which can include private or confidential information like personal details, trade secrets, or intellectual property particulars. Consequently, these models might inadvertently expose or leak such information during text generation or processing. For instance, a well-known South Korean electronics company experienced data leaks when an engineer leveraged ChatGPT to correct errors in chip code. In another incident, a different employee copied the defect detection code into ChatGPT.
These cases underline the risk: if sensitive information is shared with a public LLM, it could be incorporated into its training data and become retrievable with specific prompts. Security experts caution against this danger and advise careful consideration of the information shared with LLMs. In terms of safeguarding data, deploying Llamma on-premises presents a more secure option compared to using GPT on OpenAI's cloud service.
Prompt Injections
Prompt injection is a cybersecurity concern where hackers strategically manipulate inputs to influence the responses or actions of LLMs. For instance, cybercriminals who subtly alter queries in customer service chatbots can input normal-looking questions but embed commands that trick chatbots into revealing sensitive user data. This is known as direct prompt injection, where the attacker directly modifies the model’s prompts to access otherwise unauthorized data.
On the other hand, in indirect prompt injection, the hacker could insert malicious code into a document. When an LLM processes this document, perhaps to summarize its contents, the hidden code could mislead the LLM into generating false or harmful information.
The risks with prompt injection range from unauthorized data leaks to manipulating automated decisions – highlighting the importance of safeguarding LLMs against such vulnerabilities.
Development and Training Cost
While public LLMs have several disadvantages, establishing a self-hosted LLM introduces its own challenges, primarily financial. Developing and training LLMs, like GPT-3, which cost OpenAI over $4.6 million, require significant data and computing power, making it an expensive investment for any business.
Moreover, deploying and maintaining a self-hosted LLM involves more than just the initial investment in specialized hardware and software, which can amount to around $60,000 over five years for basic setups and up to $95,000 for scalable options. The often prohibitive costs would also include outlays for hiring a team of data scientists and support staff, building an appropriate operating environment for the LLM, and covering ongoing maintenance expenses.
Environmental Impact
Datacenters, essential for housing the servers needed for language processing models, consume a vast amount of energy and contribute considerably to carbon emissions. Models like ChatGPT have a significant environmental impact, with an estimated annual carbon dioxide emission of 8.4 tons.
Another study by the University of California highlighted the water footprint of AI models. It reported that training Microsoft’s GPT-3 model led to the consumption of about 700,000 liters of freshwater in datacenters. This quantity is on par with the water required to produce hundreds of cars. The training process generates considerable heat, necessitating large amounts of freshwater for cooling purposes.
As language models grow larger, finding ways to reduce their environmental impact will therefore become crucial for sustainable advancement. However, it is important to note that the environmental and sustainability challenges we face are not exclusive to Large Language Models (LLMs),but are prevalent across the cloud computing technologies landscape.
Collaborative Efforts to Strengthen LLMs: Addressing Flaws and Mitigating Challenges
The rapid growth and accelerating adoption of LLMs signal a transformative shift across industries and segments. It is advised to leverage LLMs cautiously in crucial projects, ensuring they undergo expert scrutiny. The models, however, remain ideal for creative tasks that do not demand the same level of focus as in mission-critical assignments.
As we move forward, it is essential to balance innovation with ethical considerations, ensuring that LLMs are developed and used in ways that benefit society while aiding businesses. Our journey toward overcoming these limitations, therefore, has to be a collective effort involving developers, users, and policymakers. The narrative should include reasons behind the creation of LLMs, examine their current status, and chart a course for their future development and integration.
By tackling these challenges head on, we can harness the full potential of LLMs to create more informed, equitable, and sustainable solutions for the road ahead.