Predictive Maintenance for Attaining Effective Transformer Health
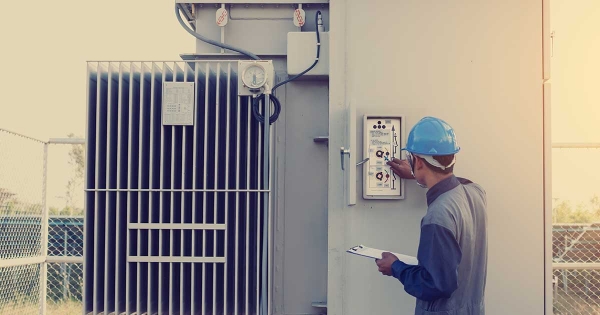
According to the futurist Ray Kurzweil “…we won't experience 100 years of progress in the 21st century—it will be more like 20,000 years of progress at today's rate.” At this juncture, when we continue to make technological breakthroughs, generating and consuming energy at an incomprehensible pace, will we remain a Type 0 civilization? It seems unlikely, if we go by the Global Energy Statistical Yearbook 2017 which says worldwide electricity production stands at a whopping 24,859 TWh. An even more alarming figure is that of the annual global transmission and distribution losses, which account for over 8% of the total power generated. Clearly, we are reaching a turning point. If we are to go by the Kardashev scale, there is an urgent need to optimize energy consumption. The first step toward achieving that would be to take a hard look at the distribution network’s health—particularly, power transformers. A vital component of the transmission grid, transformers have a finite lifespan which can be diminished or lengthened based on the level of maintenance and fault minimization. Most power distribution companies have to consider CAPEX and maintenance costs in this context. Typically, the lack of monitoring tools or dashboards make it difficult for organizations to literally visualize KPIs in order to implement an effective maintenance plan. This is further exacerbated by unexpected transformer failures that result in downtime, attracting penalties and marginalizing asset life. The need of the hour is an effective management system that leverages predictive data modeling to positively impact bottom line while achieving long-term sustainability goals.
Leveraging Analytics – Predictive Maintenance in Action
Currently, utilities are at a stage where they have the tools to survey, identify, and analyze asset health data. This diagnostic approach reveals what is wrong, leaving the operator to extrapolate a solution based on past experiences and guesswork. There is, however, a more effective way to utilize historic transformer data. With a predictive maintenance framework in place, organizations can immediately begin gleaning actionable insights from this existing mass of information. Moreover, this system grows more intelligent once the entire fleet of transformers is embedded with sensors to feed it with real-time data. A machine learning and artificial intelligence (AI) layer on top of the analytics platform then allows the system to instruct maintenance operations, informing them of the best course of action. It is hardly surprising that 25% of utilities plan to invest in sensors and cognitive capabilities to reduce maintenance costs and improve asset efficiency by 2020. Such a predictive maintenance model, supported by an IoT-driven smart network, can potentially transform the approach taken toward assessing and managing transformer health. By forecasting failure events and scheduling maintenance, repair, and overhaul (MRO) activities, it can enable these vital grid components to safely operate at maximum load and energy efficiency for extended periods of time.
Moving Closer to the Edge – Responsive Maintenance
One can argue that such a solution continues to be limited by its need for a centralized system built around the Cloud. In essence, data captured by sensors must be transmitted to a platform for processing before issues can be identified and resolved. Being sensitive assets and critical to the transmission and distribution ecosystem, transformers require rapid responses to issues for ensuring uninterrupted uptime. Since transformer fleets are extensively distributed across a geography, edge intelligence is the logical solution for exploiting this topology effectively. Based on fog computing, such a solution can extend data processing and analytics closer to where the physical components of the IIoT are. It minimizes latency by maintaining close proximity to these edge devices instead of relaying large volumes of data to a distant centralized Cloud for processing. This, in turn, amplifies performance, reducing response time to failure events and coalescing into a more compelling maintenance and operational strategy. Such a holistic approach is becoming increasingly relevant in case of transformers where even a few seconds might mean the difference between a planned, agile response and major equipment failure.