AIOps: Operationalizing Artificial Intelligence to Vitalize IT
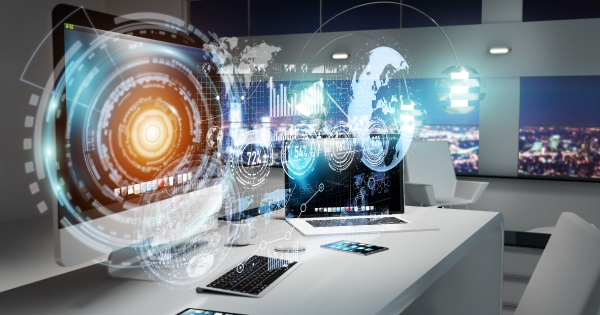
Artificial intelligence (AI) is at the cusp of revolutionizing the future of works. According to a recent study, the AI market is projected to be worth $202.57 billion by 2026, up from $20.67 billion in 2018. This tenfold increase in market size will come at a CAGR of 33.1% during the 2018-2026 period. The growing emphasis of AI, in conjunction with technologies such as computer vision, machine learning (ML), and natural language processing (NLP), will unlock new opportunities and redefine business scopes. Considering the learnings from the COVID-19 pandemic, AI will play a crucial role for enterprises to develop a future-proof, contingency-ready working model.
Among the many benefits of AI for enterprises, AIOps is arguably among the most critical one. Simply put, AIOps is the practice of applying AI to IT operations and developing a synergy between human and algorithmic intelligence. AIOps not only provides greater visibility into the performance and state of IT systems but is also a critical element of digital transformation initiatives. Operationalizing AI is imperative for enterprises to create fresh value propositions and scale quickly and seamlessly. Automated ML models help to operationalize AI across enterprises and prepare companies for contingencies of any nature.
Stumbling Blocks for AIOps
All organizations are at different stages of their digital transformation journey, and there are both industry-specific and enterprise-specific challenges to address. While companies are becoming aware of the opportunities that successful implementation of AIOps offers, they are also wary of the challenges on the transformation path and are strategizing accordingly.
The amount of data generated by smart devices is growing exponentially. This increased volume of data is posing processing challenges for enterprises. The current state of cloud computing is stifling deep learning-enabled automated algorithm creation. Similarly, the lack of skilled personnel is further compounding the problem of deploying self-learning tools and stalling machine-powered progress. To some extent, the lack of trust in self-reliant machines and processes is also a cause for the slow adoption of AIOps.
Another issue that affects organizations deciding on operationalizing AI is the lack of a thorough AI vision. There are lingering doubts about AI-based products and what they can achieve. In most cases, even when organizations operationalize AI, its effects and business impact are left to guesses. Lack of demonstration and evaluation of the decision-making capabilities of AI systems is clearly a pain point for organizations.
Similarly, there are further doubts on data privacy and security as these AI systems require access to data in order to master decision-making. Cybersecurity issues can skittle AIOps initiatives, and as a result, enterprises are sometimes too cautious. There are also issues caused by the paucity of data. This happens mostly due to the limitation of data labeling by enterprises to make them safer and more understandable.
Benefits of Operationalizing AI
While the challenges are pertinent, it is paramount for enterprises to address those challenges and further their AIOps initiative. After all, operationalizing AI is the key to the human-machine synergy and a smarter future. While talking about the benefits of AIOps, the most important one is efficient problem resolution. AIOps enables IT teams to swiftly cater to operational disruptions with the help of real-time data analysis. AIOps also helps to proactively detect system anomalies and offer a more comprehensive root-cause analysis.
Data-driven insights help AIOps to facilitate better decision making while also enhancing IT competencies. Collaboratively, all these capabilities offered by AIOps ensure improved business responsiveness and enhanced efficiencies. Here are some of the critical value propositions of AIOps:
- Situational Understanding: Data aggregation and analysis is one of the key functions of AIOps. AI-driven causality/relationship creation helps IT to understand situations better and take necessary measures to address them.
- Intelligent Alerting: Thanks to AIOps, meaningful and relevant data from the IT environment can be identified and used per the situational need. This prevents alert fatigue and business impact-based prioritization of notifications.
- Cohort Analysis: The deluge of structured and unstructured data in modern architecture makes it practically impossible for humans to sift, sort, and analyze. However, AIOps can process and analyze data being generated by multiple applications at the same time, thereby enabling real-time decision making.
- Automated Problem Solving: With the help of AI-powered root cause analysis, problems can be prioritized and addressed. Similarly, AIOps helps in swift remediation of known issues. By analyzing historical data, AIOps suggests the best approach to problem solving.
A Self-sufficient Future
The AIOps Exchange is a forum that closely monitors the progress being made in the AIOps space. In their inaugural meeting, they surveyed about 100 of the top IT leaders across industries to understand where AIOps is headed in the short and the medium terms. Almost 68% of the respondents said that their organizations already have active AIOps projects in place while about 50% of the respondents pointed out improving customer satisfaction as the primary goal for using AIOps. Similar studies have also noted that the implementation of AIOps is already showing great results, especially in the banking and finserv, retail, and healthcare sectors.
The post-pandemic world will not be the same, and the reliance on digital tools and methodologies will separate the high-flying organizations from the also-rans. Our AIOps solutions suite is aimed at catering to the IT needs of our clients and turning the challenges they face into opportunities they can optimize.
Our application performance monitoring (APM) approach focuses on taking a service-aware, user-centric approach to application performance. This helps IT teams to optimize data by processing them to identify problems and diagnose end-user experience issues. The idea is to improve overall application reliability and elevate user experience.
Our dynamic base-lining approach facilitates issue analysis and preemptive actions. Behavioral learning allows AIOps to analyze IT environments at various times and states. Knowledge from these analyses helps to derive meaningful insights on the basis of which the IT systems can generate alerts. Early detection and alerts allow for predictive incident management and prevention of potential problems that can disrupt operations. Automating the execution of standardized triage and remediation tasks is the common attribute of all our solutions.
The pandemic-caused global disruption has imparted lessons on the criticality of human-machine synergy, and AI will continue to drive that quest. The importance of AIOps can’t be overstated as enterprises ready themselves for the new normal.