Seven AI Tools that can aid Sensor Systems
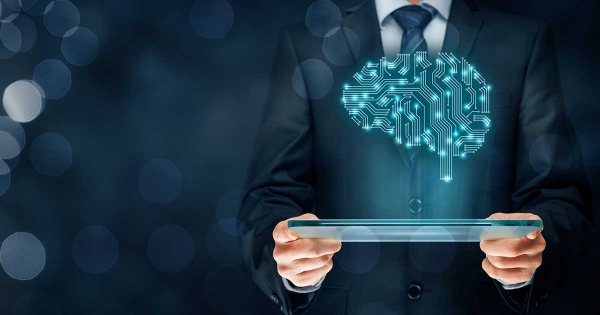
Artificial intelligence tools can aid sensor systems
At least seven artificial intelligence (AI) tools can be useful when applied to sensor systems: knowledge-based systems, fuzzy logic, automatic knowledge acquisition, neural networks, genetic algorithms, case-based reasoning, and ambient-intelligence.
Seven artificial intelligence (AI) tools are reviewed that have proved to be useful with sensor systems. They are: knowledge-based systems, fuzzy logic, automatic knowledge acquisition, neural networks, genetic algorithms, case-based reasoning, and ambient-intelligence. Each AI tool is outlined, together with some examples of its use with sensor systems. Applications of these tools within sensor systems have become more widespread due to the power and affordability of present-day computers. Many new sensor applications may emerge, and greater use may be made of hybrid tools that combine the strengths of two or more of the tools reviewed.
Seven areas where AI can help sensor systems follow.
1. Knowledge-based systems
Knowledge-based (or expert) systems are computer programs embodying knowledge about a domain for solving problems related to that domain. An expert system usually has two main elements, a knowledge base and an inference mechanism. The knowledge base contains domain knowledge which may be expressed as a combination of ‘IF–THEN' rules, factual statements, frames, objects, procedures, and cases. An inference mechanism manipulates stored knowledge to produce solutions to problems. Knowledge manipulation methods include using inheritance and constraints (in a frame-based or object-oriented expert system), retrieval and adaptation of case examples (in case-based systems), and the application of inference rules (in rule-based systems), according to some control procedure (forward or backward chaining) and search strategy (depth or breadth first).
A rule-based system describes knowledge of a system in terms of IF… THEN... ELSE. Specific knowledge can be used to make decisions. These systems are good at representing knowledge and decisions in a way that is understandable to humans. Due to the rigid rule-base structure they are less good at handling uncertainty and are poor at handling imprecision. A typical rule-based system has four basic components: a list of rules or rule base, which is a specific type of knowledge base; an inference engine or semantic reasoner, which infers information or takes action based on the interaction of input and the rule base; temporary working memory; and a user interface or other connection to the outside world through which input and output signals are received and sent.
The concept in case-based reasoning is to adapt solutions from previous problems to current problems. These solutions are stored in a database and can represent the experience of human specialists. When a problem occurs that a system has not experienced, it compares with previous cases and selects one that is closest to the current problem. It then acts upon the solution given and updates the database depending upon the success or failure of the action. Case-based reasoning systems are often considered to be an extension of rule-based systems. They are good at representing knowledge in a way that is clear to humans, but they also have the ability to learn from past examples by generating additional new cases.
2. Case-based reasoning
Case-based reasoning has been formalized for purposes of computer reasoning as a four-step process:
1) Retrieve: Given a target problem, retrieve cases from memory that are relevant to solving it. A case consists of a problem, its solution, and, typically, annotations about how the solution was derived.
2) Reuse: Map the solution from the previous case to the target problem. This may involve adapting the solution as needed to fit the new situation.
3) Revise: Having mapped the previous solution to the target situation, test the new solution in the real world (or a simulation) and, if necessary, revise.
4) Retain: After the solution has been successfully adapted to the target problem, store the resulting experience as a new case in memory.
Critics argue that it is an approach that accepts anecdotal evidence as its main operating principle. Without statistically relevant data for backing and implicit generalization, there is no guarantee that the generalization is correct. However, all inductive reasoning where data is too scarce for statistical relevance is inherently based on anecdotal evidence.
The concept in case-based reasoning (CBR) is to adapt solutions from previous problems to current problems. These solutions are stored in a database and represent the experience of human specialists. When a problem occurs that a system has not experienced, it compares with previous cases and selects one closest to the current problem. It then acts upon the solution given and updates the database depending upon the success or failure of the action.
CBR systems are often considered to be an extension of rule-based systems. As with rule-based systems, CBR systems are good at representing knowledge in a way that is clear to humans; however, CBR systems also have the ability to learn from past examples by generating additional new cases.
Many expert systems are developed using programs known as “shells,” which are ready-made expert systems complete with inferencing and knowledge storage facilities but without the domain knowledge. Some sophisticated expert systems are constructed with the help of “development environments.” The latter are more flexible than shells in that they also provide means for users to implement their own inferencing and knowledge representation methods.
Expert systems are probably the most mature among tools mentioned here, with many commercial shells and development tools available to facilitate their construction. Consequently, once the domain knowledge to be incorporated in an expert system has been extracted, the process of building the system is relatively simple. The ease with which expert systems can be developed has led to a large number of applications of the tool. In sensor systems, applications can be found for a variety of tasks, including selection of sensor inputs, interpreting signals, condition monitoring, fault diagnosis, machine and process control, machine design, process planning, production scheduling, and system configuring. Some examples of specific tasks undertaken by expert systems are:
- Assembly
- Automatic programming
- Controlling intelligent complex vehicles
- Planning inspection
- Predicting risk of disease
- Selecting tools and machining strategies
- Sequence planning
- Controlling plant growth
3. Fuzzy logic
A disadvantage of ordinary rule-based expert systems is that they cannot handle new situations not covered explicitly in their knowledge bases (that is, situations not fitting exactly those described in the “IF” parts of the rules). These rule-based systems are unable to produce conclusions when such situations are encountered. They are therefore regarded as shallow systems which fail in a “brittle” manner, rather than exhibit a gradual reduction in performance when faced with increasingly unfamiliar problems, as human experts would.
The use of fuzzy logic, which reflects the qualitative and inexact nature of human reasoning, can enable expert systems to be more resilient. With fuzzy logic, the precise value of a variable is replaced by a linguistic description, the meaning of which is represented by a fuzzy set, and inferencing is carried out based on this representation. For example, an input from a sensor system of 20 can be replaced by “normal” as the linguistic description of the variable “sensor input.” A fuzzy set defining the term “normal sensor input” might be:
normal sensor input = 0.0/below 10 widgets per minute +0.5/10−15 widgets per minute +1.0/15−25 widgets per minute +0.5/25−30 widgets per minute +0.0/above 30 widgets per minute. The values 0.0, 0.5, and 1.0 are the degrees or grades of membership of the sensor ranges below 10 (above 30), 10−15 (25−30), and 15−25 to the given fuzzy set. A grade of membership equal to 1 indicates full membership, and a null grade of membership corresponds to total non-membership.
Knowledge in an expert system employing fuzzy logic can be expressed as qualitative statements (or fuzzy rules), such as, “If the input from the room temperature sensor is normal, then set the heat input to normal.” A reasoning procedure known as the compositional rule of inference, which is the equivalent of the modus-ponens rule in rule-based expert systems, enables conclusions to be drawn by generalization (extrapolation or interpolation) from the qualitative information stored in the knowledge base. For instance, when a sensor input is detected to be “slightly below normal,” a controlling fuzzy expert system might deduce that the sensor inputs should be set to “slightly above normal.” Note that this conclusion might not have been contained in any of the fuzzy rules stored in the system.
Fuzzy expert systems (FES) use fuzzy logic to handle the uncertainties generated by incomplete or partially corrupt data. The technique uses the mathematical theory of fuzzy sets to simulate human reasoning. Humans can easily deal with ambiguity (areas of grey) in terms of decision making, yet machines find it difficult.
Fuzzy logic has many applications in sensor systems where the domain knowledge can be imprecise. Fuzzy logic is well suited where imprecision is inherent due to imprecise limits between structures or objects, limited resolution, numerical reconstruction methods, and image filtering. For example, applications in structural object recognition and scene interpretation have been developed using fuzzy sets within expert systems. Fuzzy expert systems are suitable for applications that require an ability to handle uncertain and imprecise situations. They do not have the ability to learn as the values within the system are preset and cannot be changed.
Notable successes have been achieved in the areas of:
- Cooperative robots
- Mobile robots
- Prediction of sensory properties
- Supply chain management
- Welding.
4. Automatic knowledge acquisition
Getting domain knowledge to build into a knowledge base can be complicated and time consuming. It can be a bottleneck in constructing an expert system. Automatic knowledge acquisition techniques were developed to address this, for example, in the form of IF–THEN rules (or an equivalent decision tree). This sort of learning program usually requires a set of examples as a learning input. Each example is characterized by the values of a number of attributes and the class to which they belong.
One approach for example is through a process of “dividing-and-conquering,” where attributes are selected according to some strategy (for example, to maximize the information gain) to divide the original example set into subsets, and the inductive learning program builds a decision tree that correctly classifies the given example set. The tree represents the knowledge generalized from the specific examples in the set. This can subsequently be used to handle situations not explicitly covered by the example set.
In another approach known as the “covering approach,” the inductive learning program attempts to find groups of attributes uniquely shared by examples in given classes and forms rules with the IF part as conjunctions of those attributes and the THEN part as the classes. The program removes correctly classified examples from consideration and stops when rules have been formed to classify all examples in the given set.
Another approach is to use logic programming instead of propositional logic to describe examples and represent new concepts. That approach employs the more powerful predicate logic to represent training examples and background knowledge and to express new concepts. Predicate logic permits the use of different forms of training examples and background knowledge. It enables the results of the induction process (the induced concepts) to be described as general first-order clauses with variables and not just as zero-order propositional clauses made up of attribute-value pairs. There are two main types of these systems, the first based on the top-down generalization/specialization method, and the second on the principle of inverse resolution.
A number of learning programs have been developed, for example ID3, which is a divide-and-conquer program; the AQ program, which follows the covering approach; the FOIL program, which is an ILP system adopting the generalization/specialization method; and the GOLEM program, which is an ILP system based on inverse resolution. Although most programs only generate crisp decision rules, algorithms have also been developed to produce fuzzy rules.
The requirement for a set of examples in a rigid format (with known attributes and of known classes) has been easily satisfied by requirements in sensor systems and networks so that automatic learning has been widely used in sensor systems. This sort of learning is most suitable for problems where attributes have discrete or symbolic values rather than those with continuous-valued attributes as in many sensor systems problems.
Some examples of applications of inductive learning are:
- Laser cutting
- Mine detection
- Robotics
5. Neural networks
Neural networks can also capture domain knowledge from examples. However, they do not archive the acquired knowledge in an explicit form such as rules or decision trees, and they can readily handle both continuous and discrete data. They also have a good generalization capability as with fuzzy expert systems.
A neural network is a computational model of the brain. Neural network models usually assume that computation is distributed over several simple units called neurons, which are interconnected and operate in parallel. (Hence, neural networks are also called parallel-distributed-processing systems or connectionist systems.)
The most popular neural network is the multi-layer perceptron, which is a feedforward network: all signals flow in one direction from the input to the output of the network. Feedforward networks can perform static mapping between an input space and an output space: the output at a given instant is a function only of the input at that instant. Recurrent networks, where the outputs of some neurons are fed back to the same neurons or to neurons in layers before them, are said to have a dynamic memory: the output of such networks at a given instant reflects the current input as well as previous inputs and outputs.
Implicit “knowledge” is built into a neural network by training it. Some neural networks can be trained by being presented with typical input patterns and the corresponding expected output patterns. The error between the actual and expected outputs is used to modify the strengths, or weights, of the connections between the neurons. This method of training is known as supervised training. In a multi-layer perceptron, the back-propagation algorithm for supervised training is often adopted to propagate the error from the output neurons and compute the weight modifications for the neurons in the hidden layers.
Some neural networks are trained in an unsupervised mode, where only the input patterns are provided during training and the networks learn automatically to cluster them in groups with similar features.
Artificial neural networks (ANNs) typically have inputs and outputs, with processing within hidden layers in between. Inputs are independent variables and outputs are dependent. ANNs are flexible mathematical functions with configurable internal parameters. To accurately represent complicated relationships, these parameters are adjusted through a learning algorithm. In “supervised” learning, examples of inputs and corresponding desired outputs are simultaneously presented to networks, which iteratively self-adjust to accurately represent as many examples as possible.
Once trained, ANNs can accept new inputs and attempt to predict accurate outputs. To produce an output, the network simply performs function evaluation. The only assumption is that there exists some continuous functional relationship between input and output data. Neural networks can be employed as mapping devices, pattern classifiers, or pattern completers (auto-associative content addressable memories and pattern associators). Like expert systems, they have found a wide spectrum of applications in almost all areas of sensor systems, addressing problems ranging from modeling, prediction, control, classification, and pattern recognition, to data association, clustering, signal processing, and optimization. Some recent application examples are:
- Feature recognition
- Heat exchangers
- Inspection of soldering joints
- Optimizing spot welding parameters
- Power
- Tactile displays
- Vehicle sensor systems
6. Genetic algorithms
A genetic algorithm is a stochastic optimization procedure inspired by natural evolution. A genetic algorithm can yield the global optimum solution in a complex multi-modal search space without requiring specific knowledge about the problem to be solved. However, for a genetic algorithm to be applicable, potential solutions to a given problem must be representable as strings of numbers (usually binary) known as chromosomes, and there must be a means of determining the goodness, or fitness, of each chromosome. A genetic algorithm operates on a group or population of chromosomes at a time, iteratively applying genetically based operators such as cross-over and mutation to produce fitter populations containing better solution chromosomes.
The algorithm normally starts by creating an initial population of chromosomes using a random number generator. It then evaluates each chromosome. The fitness values of the chromosomes are used in the selection of chromosomes for subsequent operations. After the cross-over and mutation operations, a new population is obtained and the cycle is repeated with the evaluation of that population.
Genetic algorithms have found applications in sensor systems problems involving complex combinatorial or multi-parameter optimization. Some recent examples of those applications are:
- Assembly
- Assembly line balancing
- Fault diagnosis
- Health monitoring
- Power steering.
7. Ambient-intelligence
Ambient intelligence has been promoted for the last decade as a vision of people working easily in digitally controlled environments in which the electronics can anticipate their behavior and respond to their presence. The concept of ambient intelligence is for seamless interaction between people and sensor systems to meet actual and anticipated needs.
Use in industry has been limited, but new, more intelligent and more interactive systems are at the research stage. From the perspective of sensor systems, a less human and more system-centered definition of ambient intelligence needs to be considered. Modern sensor concepts tend to be human-centered approaches so that the application of ambient intelligence technologies in a combination with knowledge management may be a promising approach. Many research issues still have to be resolved to bring the ambient intelligence technology to industrial sectors, such as robust, reliable (wireless) sensors and context-sensitivity, intelligent user interfaces, safety, security, and so forth.
Ambient intelligence information and knowledge gathered from sensors within an environment represents an untapped resource for optimization processes and for possibilities to provide more efficient services. The introduction of ambient intelligence technologies is still in an initial phase. However, it is promising to bring advantages in flexibility, reconfigurability, and reliability. At the same time, prices of sensors and tags are decreasing. Development and implementation of new concepts based on ambient intelligence systems in the mid- and long-term are likely. A large number of industrial companies will probably introduce different ambient intelligence technologies to the shop-floor.
On the other hand, vendors of sensors will need to equip their products with additional ambient intelligence features and utilize the advantages of ambient intelligence integrated sensors within the environment to provide new functionalities (for example: self-configuration, context-sensitivity, etc.) and improve performances of their products.
Monitoring and controlling machinery: Simple rules are being investigated that modify pre-planned paths and improve gross robot motions associated with pick-and-place assembly tasks, and rules to predict terrain contours are being developed using a feed-forward neural network. Case-based reasoning is being applied to reuse programs (or parts of programs) to automatically program sensor arrays. The combined work is already showing that automatic programming and re-programming may help to introduce environmental sensors into smaller and medium enterprises. Other projects are using simple expert systems to improve the use of sensor data in tele-operation applications.
Process monitoring and control: An expert system is being developed to assist in process control and to enhance the implementation of statistical process control. A bespoke expert system uses a hybrid rule-based and pseudo object-oriented method of representing standard statistical process control knowledge and process-specific diagnostic knowledge. The amount of knowledge from sensor arrays and sensor systems can be large, which justifies the use of a knowledge-based systems approach. The system is being enhanced by integrating a neural network module with the expert system modules to detect any abnormal patterns.
Monitoring sensor arrays: A system has been created to monitor sensors in a high recirculation airlift reactor (a process to produce clean water). Reactors can be at the edge of stability, and that requires accurate interpretation of real-time sensor data from sensors, such as: flow rate, air input, pressure, etc. A second system is interpreting data from ultrasonic sensor arrays on tele-operated mobile robots and on wheelchairs.
Fuzzy monitoring and control: A robotic welding system is being created that uses image processing techniques and a computer-aided design (CAD) model to provide information to a multi-intelligent decision module. The system uses a combination of techniques to suggest weld requirements. These suggestions are evaluated, decisions are made, and then weld parameters are sent to a program generator. The status of the welding process is difficult to monitor because of the intense disturbance during the process. Other work is using multiple sensors to obtain information about the process. Fuzzy measurement and fuzzy integral methods are being investigated to fuse extracted signal features in order to predict the penetration status of the welding process.
Neural-network-based product inspection: Two projects are using neural networks for product inspection: one is recognizing shipbuilding parts and a second is using cameras to detect and classify defects. Neural networks are useful for these types of applications because of the common difficulty in precisely describing various types of defects and differences. The neural networks can learn the classification task automatically from examples.
The first system is managing to recognize shipbuilding parts using artificial neural networks and Fourier descriptors. Improvements have been made to a pattern recognition system for recognizing shipbuilding parts. This has been achieved by using a new, simple and accurate corner-finder. The new system initially finds corners in an edge-detected image of a part and uses that new information to extract Fourier descriptors to feed into a neural network to make decisions about shapes. Using an all-or-nothing accuracy measure, the new systems have achieved an improvement over other systems.
A second intelligent inspection system has been built that consists of cameras connected to a computer that implements neural-network-based algorithms for detecting and classifying defects. Outputs from the network indicate the type of defect. Initial investigation suggests that the accuracy of defect classification is good (in excess of 85%) and faster than manual inspection. The system is also used to detect defective parts with a high accuracy (almost 100%).
Genetic algorithms to create an ergonomic workplace layout: A genetic algorithm for deciding where to place sensors in a work cell is being developed. The layout produced by the program will be such that the most frequently needed sensors are prioritized. A genetic algorithm is suitable for this optimization problem because it can readily accommodate multiple constraints.
Ambient intelligence to improve energy efficiency: Ambient intelligence and knowledge management technologies are being used to optimize the energy efficiency of manufacturing units. These benefits both the company and the environment as the carbon footprint is reduced. Different measuring systems are being applied to monitor energy use. Ambient data provide the opportunity to have detailed information on the performance of a manufacturing unit. Knowledge management facilitates processing this information and advises on actions to minimize energy usage but maintain production. Existing energy consumption data from standard measurements is being complemented by ambient intelligence related measurements (from interactions of human operators and machines/processes and smart tags) as well as process related measurements (manufacturing line temperatures, line pressure, production rate) and knowledge gathered within the manufacturing assembly unit. This is fed to a service oriented architecture system.
In our next post, we will look at hybrid techniques that bring together the best in different AI tools for effective communication, reduced errors and extended sensor life.