Reducing Cost of Quality: Calibrate, Automate, Assure
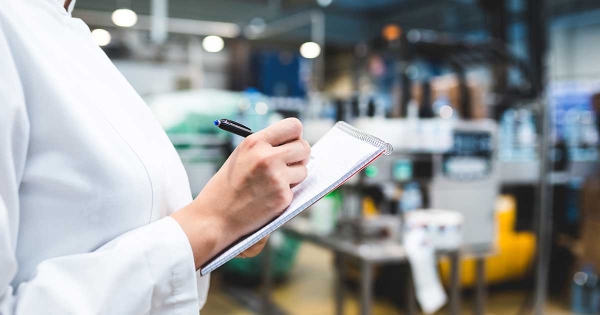
Have you read the news lately? From small and large CPG manufacturers to even automakers, a sizeable number of enterprises across industries are making headlines with massive product recalls. The reasons vary. While an Australian packaged foods company pulled its product off the shelves after tests confirmed E. coli contamination, an Irish Medtech company withdrew an entire range of insulin pumps from the market because of a flaw in design.
No matter how you assess the situation, the facts are clear and the numbers speak for themselves – the number of product recalls are going up every year with US companies withdrawing as many as 540 food products in 2016 alone. According to a joint study published by the Food Marketing Institute and Grocery Manufacturers Association, the average cost of recalling a product amounts to approximately $10 million. And that’s just the direct cost. Factor in possible litigation, declining stock value, statutory penalties, and lost sales, and you are likely to see the figure climb even higher. According to one of the leading product recall insurers, 80% of the total costs slowly begin surfacing long after the event has been dealt with. A lapse in product quality ultimately affects brand reputation and with increasing media reach, the aftershocks are likely to be much more damaging than in the past.
Accuracy without Intelligence
Quality is all about delivering standardized customer experiences that consistently meet or, ideally, exceed expectations. Yet, organizations often find it difficult to do just that. While competition pressures manufacturers into focusing on reducing cost of production and increasing throughput, improving quality practices take the back seat. Faced with dwindling margins, long ROI cycles deter them from investing in quality inspection (QI) automation. The existing installed base is aging rapidly along with the supporting legacy IT and OT infrastructure, and the lack of skilled personnel for either operating or intervening in the QI process only makes matters worse.
Quality management for manufacturing processes is bogged down by a number of other factors too. Due to the obvious lack of preplanning, various quality control data points go unrecorded and ultimately fail to support statistical analysis for gaining insights into how defects can be remediated. In some cases, even products in-process with known defects do not have a defined management procedure which indicates that the corresponding tracking, monitoring, and recording of subsystem data are not in place.
Considering the scenario, manufacturers need to start thinking about QI systematically. Apart from the general Six Sigma principles and statistical process control (SPC) that track production KPIs to ensure zero defect before a product leaves the factory, we’ll need to take a closer look at the equipment we are using for QI. Manufacturers use a range of equipment, including 3D scanners, borescopes, coordinate measuring machines (CMMs) and probes, and digital microscopes, for checking product quality at different stages of assembly. While these devices operate efficiently as separate entities, siloed inspection activities make it rather difficult to set up a unified engineering quality management system (EQMS). For this, we will need to look a little deeper and begin by converging process, production, resource, and QI data on to a single platform which can then be correlated and analyzed for insights. With emerging wired and wireless technologies like narrow-band IoT (NB-IoT) and edge sensors, it is now possible to aggregate information from traditionally disconnected sources like manufacturing execution system (MES), enterprise resource planning (ERP) systems in real time. Once compiled and statistically analyzed, the QI information can be used to inform a closed loop of continuous product improvement.
An Eye for Details
The Industrial Internet of Things (IIoT), however, promises to rewire QI processes. High-fidelity, low-cost sensors are already being installed on existing machinery to successfully support everything from predictive maintenance frameworks to real-time process control. Why not use the same principles for QI on the factory floor then?
The idea has found a place in the oil & gas (O&G) sector – in collaboration with technology partners, companies are using an installed base of edge sensors like thermal scanners, ultrasound imaging, and data analytics to facilitate real-time quality control. Wireless sensors communicate with programmable logic controllers (PLCs) for making quick changes to inspection processes based on insights gleaned from captured data. Add to that an updated supervisory control and data acquisition (SCADA) system, and the QI process will factor in contextual quality attributes to flag defects in a product as soon as they appear during the manufacturing process.
And that’s not all; major breakthroughs in high speed machine vision-based QI systems are making big waves. Rather than depending on a human operator for in-process inspection and quality control – a task made increasingly difficult by an explosion in product variety – manufacturers can utilize cameras driven by computer vision algorithms to detect defects immediately and identify root causes of failure. It increases the efficiency of the anomaly detection process and equips it to handle hundreds of units in seconds, rather than hours. In addition, it enables manufacturers to identify and resolve production disruptions before expensive delays occur. The upcoming generation of such systems is slated to be four times faster than their predecessors and will use rugged smart cameras with fully integrated liquid lens capable of detecting objects as small as 2 mm.
Going a step further with computer vision-based technology for QI, we are likely to discover that artificial intelligence (AI) will become a key component of the process in the near future. One of the largest names in cognitive computing research recently announced that they will be using the same technology that beat Jeopardy! champions Brad Rutter and Ken Jennings on the factory floor. Instead of just using ultra high definition cameras for visual inspection, the cognitive system will identify defects by matching patterns to images of defects that it has previously encountered while continuously learning from human assessment of the defect classifications in the images. This will ultimately reduce inspection time by 80% and manufacturing defects by 7-10%.