Integrated Asset Management Systems: The Brain behind Intelligent Asset-Intensive Industries
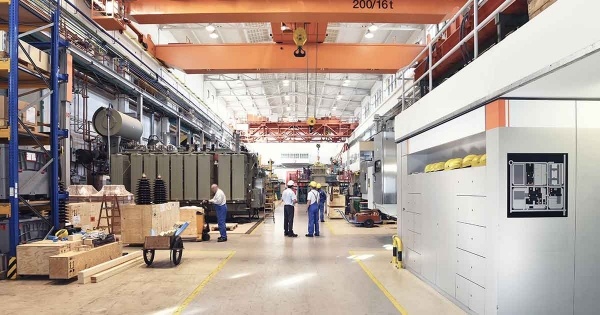
Asset-intensive industries often face overwhelming complexities involved in equipment management. Challenges like unstructured maintenance planning and poor inventory management often lead to increased capital and operational expenditure. Fragmented asset management solutions, along with the lack of a unified database, further exacerbate the situation.
In the O&G industry, where one of the main causes of pipeline malfunction is aging infrastructure, these challenges could be especially costly. In the case of the oil and gas sites in Pennsylvania, faulty equipment led to leakages of 520,000 tons of methane annually, causing wastage of nearly $68 million worth of energy resources. This shows that integrating the management of all assets is essential for optimizing resources and eliminating environmental damage.
Integrated Asset Management: Consolidating Silos and Building a Sustainable Network
Integrated asset management (IAM) systems draw inspiration from one of the most efficient connected systems known to us: the human brain. Similar to the network of neurons in the human body, an IAM system digitally connects all the assets in a facility. This allows the system to focus the entire asset lifecycle around information. But, as with any data-centric process, IAM systems are only as good as the information they work with. Since organizations rely largely on unstructured data sources like document hard copies, files, and vendor documents, IAM systems need to follow a robust data strategy.
In order to accurately capture asset data and collate them on a single integrated data platform, these systems need to effectively digitize data and ensure quality data migration. Additionally, the information management platform needs to support multidimensional data inputs across greenfield and brownfield projects. However, to create a truly connected platform, the system needs to regularly update and map the reference data library. This, in turn, simplifies data recovery and analytics.
As a result, plants and facilities have real-time visibility into asset health and performance. Consequently, this helps improve overall equipment efficiency (OEE). The concept of asset integrity can optimize asset operations and management services with various new-gen technologies. Such an approach can facilitate the development of new business models like value-based managed services.
With IAM tools, analytics on the engineering information status helps improve safety, reliability, operations, and timely handover. Quality, consistency, and redundancy issues can be eliminated by collaboration and integration throughout the asset lifecycle.
A Future of Truly Connected Facilities
IAM systems consolidate all business applications across the value chain, including enterprise asset management (EAM), enterprise resource planning (ERP), manufacturing execution systems (MES), human resource management systems (HRMS), and finance management systems. Data aggregation plays an important role in this aspect of integrated asset management. By capturing data from the various stand-alone applications and leveraging big data analytics to generate insights, data aggregators aid in the development of business strategies and making informed decisions.
Additionally, with advancements in artificial intelligence (AI), asset-intensive enterprises have the opportunity to collect large amounts of operational data from their IoT-enabled assets and enable improved predictive asset management through machine learning. In the case of an O&G major, an Israel-based startup designed a platform that incorporated deep learning and machine learning to study six months of historical sensor data from a broken-down oil well to help the organization reduce unplanned downtime for its other machinery. The platform leveraged data analysis and machine learning algorithms to establish a baseline reading. Using the numbers, the system learned to identify pressure and temperature readings that indicated imminent failure.
This instance shows that AI can enable IAM solutions to further optimize equipment health and create a productive plant environment. In addition, it underlines the potential to make what we have called the brain of a facility more experienced and richer with time.
Cognitive learning-based solutions extend the capabilities of asset data management when applied at an early stage of the asset lifecycle. For instance, in the O&G industry, combining cognitive learning with subsurface data management can reduce the probability of failure of the field project. Additionally, it can improve exploration efficiency and lower business risk and costs.
Undoubtedly, IAM solutions hold bigger opportunities for CEOs of asset-intensive industries, who map their productivity metrics with asset utilization rather than revenue and profit. Many plant operators have already responded by adopting asset management systems and reaping gains from productivity improvement and equipment uptime. This has lent momentum to the asset management system market which is poised to grow at a CAGR of 15.17% between 2016 and 2022. We have both the need and the resources today to attain greater asset efficiency. Early adoption of the IAM solution is recommended to achieve overall plant efficiency and gain a competitive edge in the market.