Envisioning a Safer Workplace with Predictive Analytics
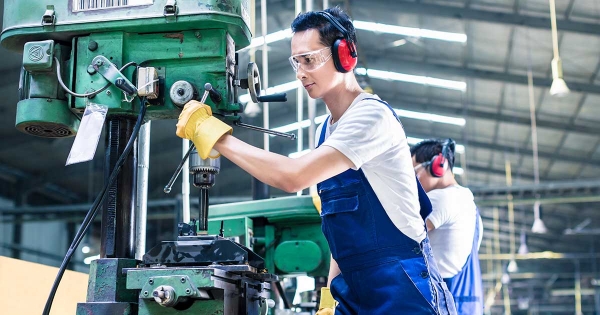
On August 5, 2017, 31-year-old Daniel Springer – a contract miner of Independent Mining Services (IMS) — sustained serious injuries while performing a routine maintenance drill at the Goonyella Riverside Mine in Queensland. Sadly, a couple of days later, he passed away leaving behind his wife and son.
Daniel is not the only one to have died due to a horrific workplace mishap. The International Labour Organization (ILO) reveals that every day, 6,300 people die because of occupational hazards or work-related diseases, taking the overall toll to an astonishing 2.3 million deaths per year. These figures don’t just prove that workplace accidents are rising; they also expose major loopholes in workplace safety and risk management.
Over the past few years, companies across the globe have made significant investments and implemented several procedural and behavioral measures to reduce the chances of workplace mishaps. In fact, there have been instances when countries such as China have conducted full-scale nationwide reviews of workplace safety. But many of these attempts have been in vain. The situation begs a very important question–where are we going wrong?
Taking a Leap–From Prevention to Prediction
Conventional workplace safety operations essentially involve preventive measures like behavioral-based safety and strategic incident counter procedures that help in promoting behavioral awareness to evading accidents. But these measures are often found wanting when it comes to predicting an accident and dealing with the root cause of its occurrence.
The key to bolstering workplace risk management initiatives may very well lie in strategically leveraging data and analytics. The advent of Big Data has vested unprecedented power in the hands of companies who seek to make more accurate predictions based on historical patterns.
Rapid innovation in sensor technology promises to further help companies gather streams of real-time operational data to identify potential threats arising from critical ‘causal factors’ such as poorly maintained machinery. By leveraging richer datasets that were previously inaccessible, risk managers can work on developing a robust safety model. This could involve a combination of predictive analytics with other concurrent technologies, including machine learning. The oil & gas and mining sectors will be among the beneficiaries of a model like this as equipment malfunction is a major reason for occupational casualties and injuries in these domains.
Predictive models for these purposes are already gaining traction. For instance, a team of researchers from the University of Saskatchewan and Chevron are conducting a series of tests at the Department of Energy’s SLAC National Accelerator Laboratory to closely evaluate various forms of sulfur present in crude oil. By employing advanced X-ray techniques, the team is developing a new analysis tool that can precisely predict how the harmful sulfur compounds could corrode equipment. These solutions can prove particularly effective in highly active oil belts like Saskatchewan where hazardous levels of hydrogen sulfide (H2S) have caused serious injuries and even death.
Making Smart Workplace Safety a Reality
Identifying potential accidents and causal factors with predictive analytics generally involves segmenting critical incident data and determining minor hotspots that can lead to mishaps. The key to locating the causal factors, however, also lies in monitoring other variables like vehicle telemetry, weather, process and equipment operation, socio-demographic, and geospatial data. This is where smart analysis makes an entry.
Organizations can exploit hi-tech yet inexpensive media like cameras and wearables, along with sensors to sift through occupational data and identify hidden patterns. For instance, a renowned truckload carrier teamed up with a driving performance solution provider to integrate a smart video-based safety platform in all trucks of its entire fleet. The platform analyzed video data transmitted by a camera that was embedded within each vehicle in order to identify the root cause of an incident. In other cases, it offered predictive insights on specific driver habits and other potential threats that could potentially cause accidents.
As predictive data-driven safety approaches start evolving as the new norm, organizations will have little choice but to upgrade their strategies. Only then can they improve safety outcomes and lower costs of workplace safety initiatives.