Decentralizing Computing Power – Bringing Intelligence Closer to the ‘Edge’
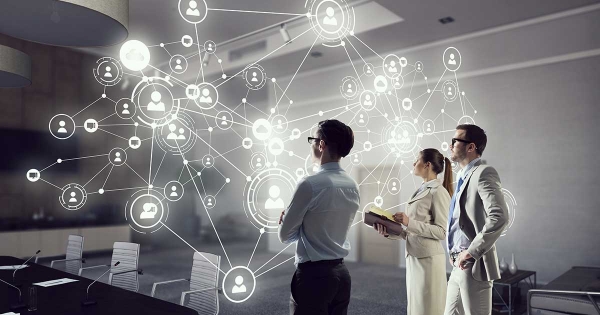
There’s often a sense of ambiguity around new tech trends. No matter how promising they sound, there will be a pool of critics trying to disregard the possibilities they bring. Consider Cloud computing as an example. Back in 2008, many industry bigwigs dismissed the trend as a fad and yet here we are – almost a decade later – watching Cloud computing being taken to the edge.
Edge Computing – Toward Advanced On-Device Processing
Cloud services have long helped companies simplify and secure their data aggregation and processing functions. But, with time as processes, devices, machines and assets became more connected and distributed, companies realized that there was a need for more immediate intelligence from data closer to the source. This is where edge computing made its entry.
With this on-device computing approach, companies can reduce latency for applications, lower their dependencies on the Cloud, and better manage huge data sets generated by the Internet of Things (IoT). It is for this reason edge computing is fast making its way into manufacturing, which now needs enormous computing power to drive its smart factories.
Edge computing can help the manufacturing fraternity combat a range of challenges, including equipment breakdown and unplanned downtime. For instance, intelligent temperature monitoring sensors in factories– network, computing and storage capabilities–can be programmed to record temperature changes in the immediate surroundings. Should there be a fire, these programmed devices can self-trigger sprinklers, send a message to the fire department, and shut down the electrical system in the factory to prevent an explosion.
A scenario such as this would require machine-to-machine (M2M) edge computing to decrease network latency and deliver real-time control and monitoring. Historically, devices at the edge only ‘knew’ how to locally collect data and transmit it to a remote server. With the advent of artificial intelligence (AI), edge devices can be equipped with machine learning capabilities to self-learn and perform actions instead of waiting for a response from a central computer.
Industrial controllers like programmable logic controllers (PLCs) in an electronics manufacturing plant can be ‘taught’ to detect an abnormality, analyze the issue, and action remedial measures to enable seamless production. The PLCs can act as edge nodes with machine learning capabilities to actuate prescriptive and predictive maintenance, without any IT or human intervention.
Optimizing Remote Processes with Greater Agility
In difficult-to-reach locations, real-time decision making is imperative for optimizing processes, saving infrastructure from major disasters, and cost management. Offshore oil platforms, for instance, are places where real-time decision making is a major challenge.
Data pertaining to oil production, drilling safety, weather monitoring, etc. continues to be generated in real-time from the supervisory control and data acquisition (SCADA) system of each platform, at the rate of about one to two terabytes daily. Sending this amount of data directly to the Cloud and expecting real-time processing requires consistent and extremely high network bandwidth, which at an offshore site like this is not always possible.
A better approach is to process the data and churn out intelligence on-site, relieving the network from unwanted latency issues. Recently, a fault tolerant computer server and software manufacturer launched a virtualized, self-protecting edge computing platform which is specifically designed for industrial control system environments. The platform comes embedded with zero-touch computing properties and is expected to simplify a range of remote management activities such as Cloud-based health monitoring, automated site and data recovery.
Combining AI with Edge Computing – A Match Made in Heaven
Together, AI and edge computing can foster unprecedented innovations in sectors like automotive where assembly line operations are still largely scattered. From operating conveyor belts to managing supply chain operations, there are several areas where there remains a need to run real-time machine learning (ML) at the edge.
Any delay in data processing on the Cloud premise can cause total network disruption in a company, leading to operational downtime. AI processors – chips that can execute advanced ML algorithms can help in alleviating this shortage of intelligence at the edge. The market for this is still in its nascent stages but the results so far are promising.
Recently, a renowned chip maker managed to embed a high-end machine vision processor into a smart plug-and-play USB stick. Companies looking to implement AI technologies within physical products or machines can leverage this USB stick to expediently manage neural networks locally.
Moving to the edge doesn’t nearly spell doom for traditional data centers. Much rather, it opens up an opportunity for them, as companies will have to tap into the capabilities of the Cloud, data centers and field devices collectively to strike the right balance between innovation, efficiency and cost.